Video-assisted diagnosis mainly does two things:
1. Medical knowledge map construction;
2. Diagnosis based on disease.
Today we will analyze how to use AI technology to identify and assist in the diagnosis of esophageal cancer.
Esophageal cancer is one of the five major malignant tumors in the world, and my country is also a high-incidence area of esophageal cancer. The goal of this project is to determine whether a patient may have cancer through images.
The overall process of the project is as follows:
1. Collecting data sets: The endoscopic probe generally enters the patient’s nasal cavity, then passes through the throat and esophagus, and finally reaches the stomach. When we collect esophageal data, we may introduce a large amount of non-esophageal data.
2. Data labeling and model establishment: Then distinguish these data, use the esophageal discriminant model, and only retain the esophageal data. Then send the esophagus data to the next model. This model only does one thing, which is to distinguish the normal esophagus from the abnormal esophagus.
3. Image analysis: After distinguishing, send the diseased esophagus data to the next link to determine whether the image represents cancer or inflammation.
The whole process can be roughly divided into these three stages, and then I will briefly introduce the difficulties of each stage.
Few image data sets and different performance
Compared with the amount of data of hundreds of thousands, millions, or even tens of millions of data in common image classification tasks, the amount of data in medical imaging is very small. At the same time, the apparent changes of the esophagus are very complicated due to the difference in equipment parameters, the doctor’s photographing technique or shooting angle, and the brightness of the light.
So, how can we get a reliable and stable model under such conditions?
Use Feature map. Feature Map is convolved by the convolution kernel. You can multiply the original image with the convolution kernel in various situations to get various feature maps. You can understand it as you analyze the picture from multiple angles. Different feature extraction (cores) will extract different features. The purpose of the model is to solve an optimization to find the best set of convolution kernels that can explain the phenomenon.
In the same layer, we hope to get a description of a picture from multiple angles. Specifically, we use a variety of different convolution kernels to roll the image, and get responses from different kernels (the kernel here can be understood as a description), as The characteristics of the image.
Their connection lies in forming the description of the image on different bases at the same level. The lower core is mainly some simple edge detectors (also can be understood as physiological simple cells).
After getting the esophageal data, how to determine whether the esophagus is a healthy normal esophagus or a diseased esophagus?
This question is similar to the previous question and is also a discriminant model.
What is the difference between them?
When we judge whether an esophagus is abnormal, we only need to find a diseased area to show that the esophagus is abnormal.
But conversely, in normal images, it cannot be said that finding a normal feature means that the esophagus is normal. It can only be said that we did not find abnormal features in this image, it may be normal.
Therefore, between normal and abnormal features, we are more inclined to extract lesion features and suppress normal features.
How did we do it?
Both the diseased and normal cases will go through the neural network to obtain the feature vector. For this vector, we hope to highlight the abnormal features as much as possible, and make the normal features approach zero.
How do we model this information into the model?
We remodeled the model, and the final accuracy rate was about 97%.
The previous model is relatively simple. The third model is mainly to distinguish inflammation and cancer. It is different from the first two problems.
Under normal circumstances, the image of the diseased esophagus will be accompanied by some features of inflammation.
Our judgment of cancer is often obtained through an area with a particularly small texture, so we need to extract more refined features. It is better to have many experts mark out the lesion area very rigorously, so that we only need to identify this area.
This label is very large, so the data is extremely scarce. We don’t have annotated data for cancer areas, but we want to get very refined features. How to solve this contradiction?
Fortunately, although we cannot obtain very accurate images of the lesion area, it is relatively easy to know whether an image contains cancer, because we only need to associate it with the case. In this way, we can get the global label of the image more easily.
If an image contains cancer, there must be one or several areas that contain cancer features. In other words, if we divide the image into several patches, there must be one or several patches containing cancer features. Based on this kind of thinking, we adopted a multi-sequence learning method. The inner idea of this method is very simple, that is, the image is divided into several patches, and then each patch is modeled to determine the probability of cancer in this patch.
We finally use the patch with the highest probability of cancer in all patches as a label for whether the image contains cancer.
In the process of doing this, we will gradually accumulate accurately labeled data, which is very small and not enough to virtualize a model. However, the features in the image are the most accurate and are manually checked and annotated.
How can we strengthen this small amount of accurate data into cancer identification?
This is a very interesting problem. If we can solve this problem, we can continue to improve even with a small amount of standard data.
The multi-task learning method is mainly used here. This method needs to complete two tasks:
1. Establish a supervised learning task based on the data marked with lesions;
2. For the data without the lesion area annotation, establish the aforementioned multi-sequence learning task.
The two models share the feature extraction network, which must meet two major tasks at the same time, so that the accurately labeled features can be enhanced into cancer recognition.
The above is a brief introduction to our esophageal cancer project, and here is a brief introduction to some of our work in assisting diagnosis.
What is the purpose of auxiliary diagnosis?
We hope that the machine will eventually be able to diagnose diseases like clinicians.
Before introducing the auxiliary diagnosis project, let’s take a look at how a doctor or an ordinary student grows into an expert: a student has learned a lot of professional courses from the beginning of enrollment, and read a lot of professional medical literature. Can accumulate a certain degree of medical knowledge.
When the medical knowledge reaches a certain level, he can go to the hospital for internship, and the clinician will combine some real cases to guide him to learn diagnostic skills.
When we have these skills, we can become an ordinary doctor. The doctor can see a large number of patients, learn a lot of experience, and become an expert after having enough experience.
The growth process of machines is roughly similar to that of humans.
We can divide it into three stages:
1. The construction of medical knowledge graph, which is the process of machine learning knowledge;
2. The ability to learn diagnosis after having knowledge, that is, to establish some models of disease discrimination;
3. Allow the machine to continuously improve the diagnostic level during the game with the expert, gradually approaching or even surpassing the expert.
In the process of constructing the medical knowledge graph, we must first process the text data. Text data is divided into two categories, one is semi-structured data, and the other is unstructured data.
Here I give an example to illustrate how we can turn unstructured text into structured text, which is a form that computers can understand.
We can divide the medical history into several parts: the condition of the disease, the treatment history of admission, the basis for admission, etc.; after dividing the medical history into such pieces of information, each type of information is refined and extracted; after extraction, Unstructured text becomes structured text that the computer can understand; we will convert this information into a medical knowledge graph and store it in the computer, so the computer learns this knowledge.
The above is the construction process of the medical knowledge graph.
In the second step we will have a diagnostic model.
The diagnosis process is like this. First, the condition described in human language is transformed into structured knowledge that the computer can understand. With structured knowledge, the machine can understand the person’s situation and push the knowledge to the disease diagnosis model. The model will give a list of diseases. The process of the diagnosis model is roughly like this.
Let’s look at an example of disease understanding.
Some basic information can be obtained by understanding the patient’s condition through technology, including gender, age, active description of the person, current medical history, and past history.
The active description will mention the symptoms and duration, and even some more complicated information, such as what the saliva looks like, whether the cough is phlegm. This information will be portrayed in detail, and the medical history will be drawn according to the aforementioned model to complete the understanding of the condition.
After understanding the condition, input it into the diagnosis model.
The diagnosis demo consists of several parts: a description of the condition in human language, a structured indication of the condition is obtained after understanding the condition, and then the result of the machine diagnosis is obtained, and 5 results are given from high to low according to the probability.
We also leave an interface for doctors, where they can score the diagnosis results and feed the results back to the model through the scores.
Through the interaction between the doctor and the machine, the model can be iterated better and better.
We selected about 100,000 real cases in the laboratory data for testing. The agreement between TOP1 results and doctors is about 92%, and TOP3 is 90%, but this model needs more clinical cases for verification.
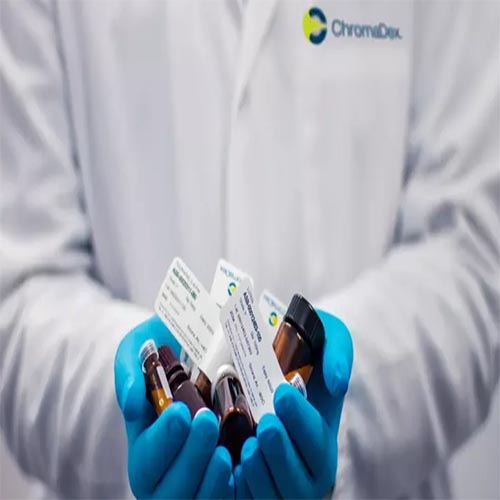